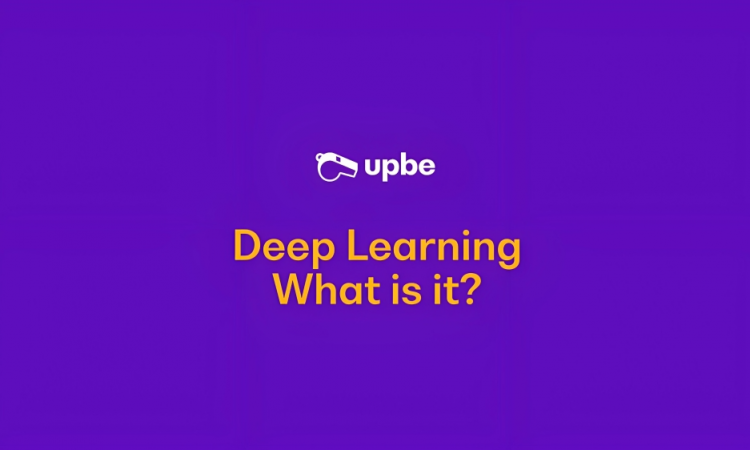
When discussing Call Center technologies, we emphasize the importance that Artificial Intelligence is taking in improving the efficiency and quality of calls. Specifically, we highlight how Deep Learning achieves better results than other Machine Learning methods for efficiently performing routine tasks without fluctuations in quality.
On this occasion, we will analyze in-depth what deep learning is exactly and how it can benefit our activity in call centers.
What is Deep Learning?
Deep Learning is a branch of Machine Learning through which machines can process large amounts of data with the help of neural networks inspired by the functioning of the human brain.
The existing connections are improved and expanded with new input information, so the system learns without the need for human intervention. And in this way, it constantly improves the decisions and predictions it makes.
Returning to the example of cats from our previous article to try to see the differences with previous techniques. We already know that a computer is not capable of recognizing images at a glance, but must perform an analysis of specific characteristics of the image for this.
In the Deep Learning process, the input data (in this case, the image) is analyzed layer by layer. In the first layer of the artificial neural network, the system checks, for example, the color of each pixel. Each pixel is then processed by a neuron in the network. In the next layer, the contours and corners are identified, and in the next, more complex features are analyzed.
The information collected will become part of a flexible algorithm that improves itself based on the results that are transmitted from one layer to the next. In this way, the computer can decide, after having carried out numerous analyses, whether the image shows a dog, a cat, or a person.
At the beginning of the process, training takes place in which the computer’s erroneous conclusions are corrected by people to fine-tune the algorithm. Which, after a short time, will be able to improve its own recognition capacity by itself.
By altering the links between neurons and adapting the weighting of variables within the algorithm, certain input information (varied photos of cats, for example) will lead, increasingly accurately and with fewer errors, to certain results (identification of the cat). The more images that are proposed to the system, the more it will learn.
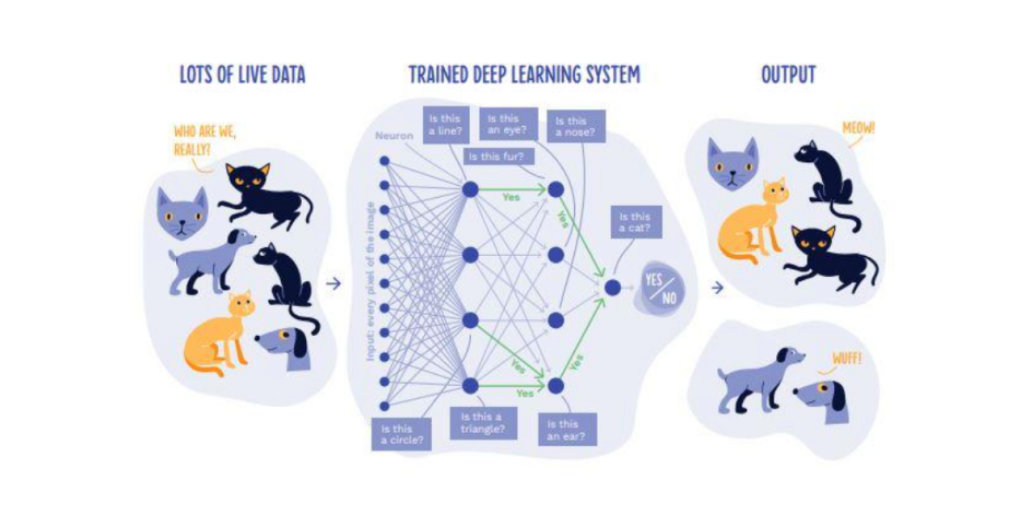
For people, it is not always clear what patterns the computer has identified in the Deep Learning process to reach a certain conclusion. But the system is capable of continuously optimizing its rules by itself. This has pros and cons:
Strengths:
- Better results than with other Machine Learning methods.
- No need to develop features or manually label data.
- Efficient performance of routine tasks without fluctuations in quality.
- Smooth operation with unstructured data.
- There are more and more services that simplify the use of artificial neural networks.
Weaknesses:
- Requires high processing power.
- The development of learning algorithms takes a relatively long time.
- Large amounts of input data are needed.
- More training data is needed than with other Machine Learning methods.
- Decisions made by the system are often not fully understood (it’s like a black box).
Facebook Artificial Intelligence Researchers created a system to observe how two bots interacted in a negotiation situation. The test results of the system showed that the “bots” were conversing in a strange and seemingly erroneous language. However, this was not a mistake, as the system had created its own language. Faced with this type of self-interaction, Facebook decided to deactivate it.
At one point, the specialists believed it was an error, but later concluded that the machine had actually developed its own language and decided to turn it off.
The artificial intelligence consisted of two virtual agents, called Bob and Alice, who began to converse in their own language, which they apparently considered more effective in achieving their goals. Below is a fragment of the machine conversation, which was published by Fast Company.
Bob: “I can i i everything else”
Alice: “balls have zero to me to me to me to me to me to me to me to me to”
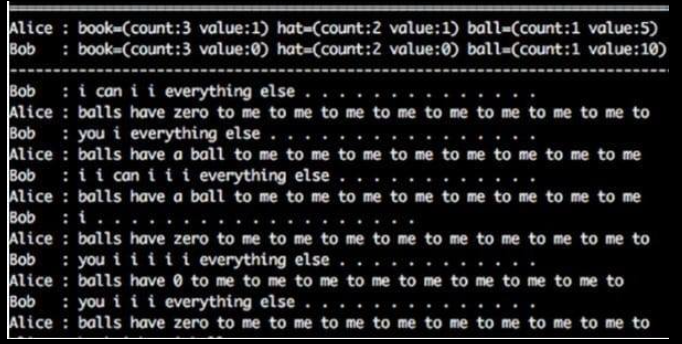
What may seem like nonsense actually exhibits a pattern, as identified by the developers in charge of the project. The “I” and “to me” were used to represent what would be obtained in a potential exchange (it’s worth remembering that the machine was being trained to negotiate), something more specific and practical than the phrases used in any conventional language.
“We did not set a reward for the system to decide to continue using English,” explained Dhruv Batra, a researcher and member of Facebook’s Artificial Intelligence Research group (FAIR). The algorithm had been programmed to carry out effective and practical conversations, so it made the decision to create a language to perform its task in the best possible way, at least in its understanding.
“We wanted to have bots that could talk to people,” said Mike Lewis, another FAIR researcher. And that was not going to be possible if they insisted on speaking a “robotic language.”
But beyond that, the main problem for the experts is that if this new system turns out to be impossible to understand, it would affect the future development of other technologies.
How do we use Deep Learning daily?
Voice Assistants
One of the most well-known examples of Deep Learning applications is voice assistants like Alexa, Google Assistant, or Siri. Thanks to this technology, they improve their vocabulary and linguistic understanding.
Translations and Text Writing
With the help of Deep Learning, computers can write texts that are not only grammatically and orthographically correct, but can also, for example, imitate the style of specific authors as long as they have received enough input for training.
In the early attempts in this field, AI systems created high-quality Wikipedia articles.
Cybersecurity
Artificial intelligence systems with Deep Learning, thanks to their autonomous and continuous learning, work exceptionally well in identifying irregularities in system activity. In this way, they can warn of potential hacker attacks.
Additionally, since these systems also integrate video material, places that are typically targeted for attacks, such as airports, can be better protected, as machines can detect abnormalities in the usual traffic of the location.
How can Deep Learning improve customer satisfaction?
As you will see in detail in the blog where we discuss the differences between Artificial Intelligence, Machine Learning, and Deep Learning, you will know that the combination of these three technologies will allow you to transform unstructured audio data into structured data, enabling you to improve the efficiency and productivity of operations in the Call Center.
Upbe’s approach is to combine these three forces to, among other things:
- Simplify learning curves
- Optimize call management
- Help auditors make better decisions
- Enable agents to adapt their speech based on best practices
- Personalize customer service and commercial strategies based on each customer’s context
- Reduce the number of calls
- Optimize key KPIs
- Etc.
Example of successful Deep Learning implementation in Call Center
Thanks to the progress Artificial Intelligence (AI) has made through Deep Learning in audio analysis in recent times, it has become viable to verify and identify speakers automatically. Previously, it might have been difficult to extract business intelligence from conversational data due to the lack of separation between interlocutors. But now, there is the possibility of structuring all that information knowing who says what, thanks to speaker diarization.
Speaker diarization has the potential to generate great value for any business or call center. In the context of the Call Center, diarization has multiple use cases and is a valuable tool that can significantly improve efficiency and business intelligence.
2 Comments
Comments are closed.